Unlocking the Power of Image Annotation for Machine Learning
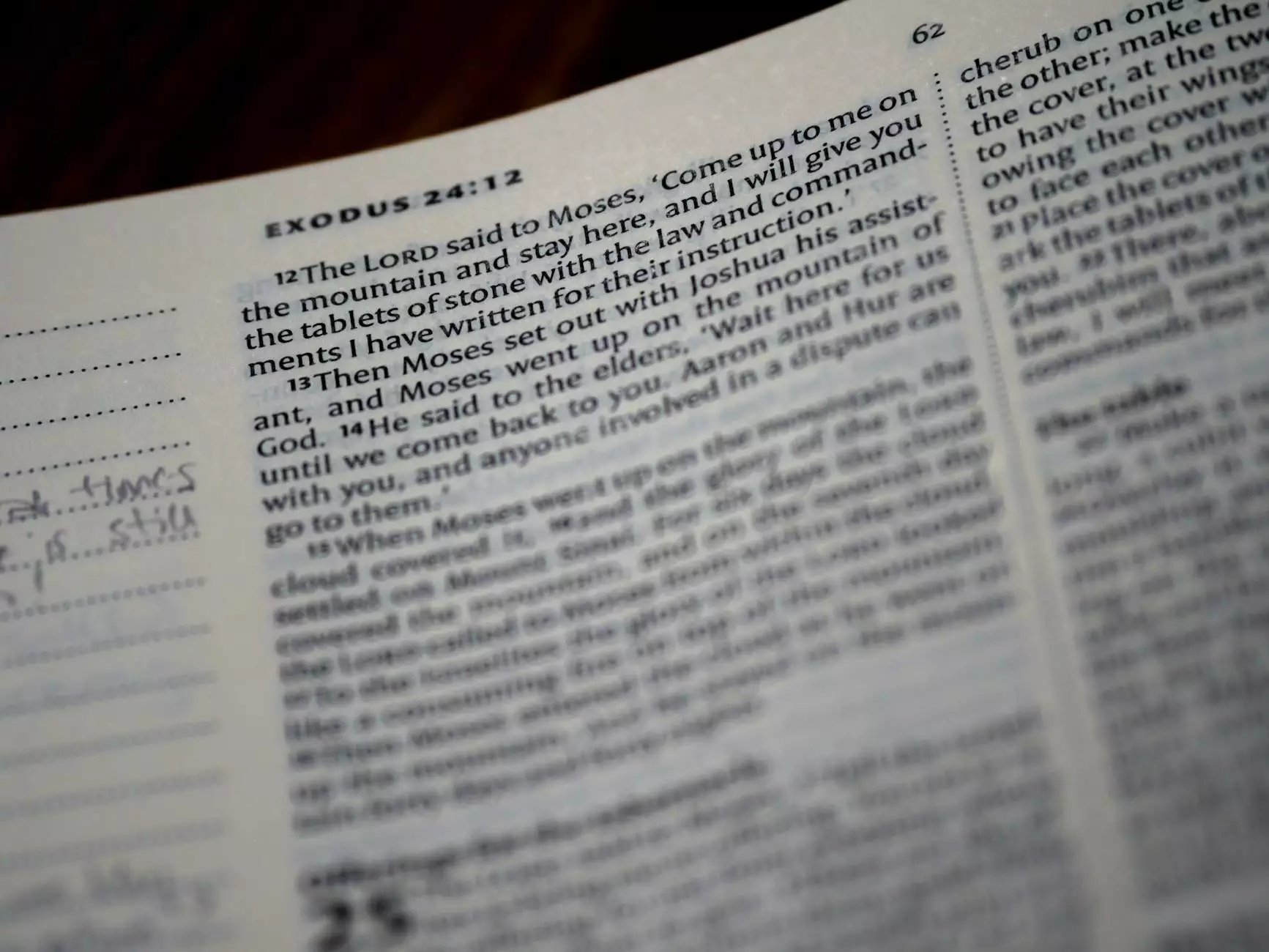
Image annotation for machine learning is a crucial process that enables artificial intelligence (AI) and machine learning (ML) algorithms to understand visual data. As businesses strive to leverage data-driven insights, the significance of image annotation cannot be overstated. This article delves into the various aspects of image annotation, its applications, benefits, and how it can revolutionize business operations, particularly through platforms like KeyLabs.ai.
What is Image Annotation?
Image annotation refers to the process of labeling images with informative tags or descriptions. These annotations provide context to the images, enabling machine learning models to learn from visual data effectively. The accuracy and quality of these annotations directly affect the performance of AI systems.
The Importance of Image Annotation in Business
In today's data-centric world, businesses across various industries are harnessing the power of AI for improved decision-making. Here’s why image annotation for machine learning is pivotal:
- Enhanced Data Quality: High-quality annotations lead to better model training, resulting in more accurate predictions.
- Scalability: As businesses accumulate vast amounts of data, efficient annotation processes allow for scalable AI applications.
- Competitive Advantage: Companies utilizing advanced ML capabilities can outperform their rivals in various areas such as customer service and operational efficiency.
- Versatility: Image annotation can be applied to numerous sectors, including healthcare, automotive, retail, and security.
Types of Image Annotation Techniques
There are several methods for conducting image annotation, each with specific applications:
- Bounding Boxes: This method involves drawing rectangles around objects in an image. It's widely used in object detection tasks, such as identifying vehicles in traffic images.
- Semantic Segmentation: Unlike bounding boxes, this technique assigns a class label to each pixel in an image, providing a detailed understanding of object boundaries.
- Image Classification: This method involves categorizing images into predefined labels, which helps in organizing visual data.
- Keypoint Annotation: This technique involves marking specific points on an object, crucial for tasks like pose estimation in robotics.
- Polygon Annotation: Often used for irregularly shaped objects, polygon annotation provides a more accurate outline than bounding boxes.
Applications of Image Annotation for Machine Learning
The applications of image annotation for machine learning are extensive. Here are some prominent areas where it plays a critical role:
1. Healthcare
In healthcare, image annotation is vital for training models to identify diseases from medical imaging. Annotating X-rays, MRIs, and CT scans helps in creating predictive models that assist radiologists in diagnosing conditions.
2. Autonomous Vehicles
For autonomous vehicles, precise image annotation is necessary for object detection and road sign recognition. By leveraging annotated images, machine learning algorithms can make informed decisions on the road.
3. E-commerce
In e-commerce, image annotation helps improve product searches and recommendations. Accurate tagging of product images allows for better categorization and more relevant search results for users.
4. Surveillance and Security
Surveillance systems utilize image annotation to enhance security measures. By labeling behaviors and objects in images, businesses can deploy AI systems that detect unusual activity in real-time.
Key Features of a Robust Data Annotation Platform
When selecting a data annotation tool or platform, consider these essential features to ensure effectiveness:
- User-Friendly Interface: An intuitive UI makes it easier for teams to manage and execute annotations efficiently.
- Collaboration Tools: Features that promote teamwork can enhance the annotation process, ensuring consistency and quality across the board.
- Scalability: As your image dataset grows, your annotation platform should be able to scale accordingly without compromising speed or quality.
- Quality Assurance Mechanisms: Built-in QA processes help to maintain high standards in data labeling, ensuring that machine learning models receive the best data possible.
- Integration Capabilities: A good annotation platform should easily integrate with your existing workflows and tools to streamline the data pipeline.
How KeyLabs.ai Revolutionizes Data Annotation
KeyLabs.ai is at the forefront of providing advanced data annotation solutions tailored to meet the needs of businesses aiming to excel in AI applications. Here’s how it makes a difference:
1. Comprehensive Annotation Services
KeyLabs.ai offers a wide range of annotation types, ensuring that businesses can find the right solution for their specific needs. Whether it’s bounding boxes, semantic segmentation, or polygon overlays, they provide expert services to cover all areas.
2. AI-Powered Automation
Utilizing AI-driven tools, KeyLabs.ai enhances the image annotation process, reducing the time and effort required while increasing accuracy. This not only accelerates project timelines but also optimizes resource allocation.
3. Dedicated Quality Control
KeyLabs.ai implements robust quality control processes to ensure that the data delivered is of the highest quality. These mechanisms involve multiple review stages and feedback loops to guarantee precision in annotations.
4. Customizable Solutions
Every business is unique, and KeyLabs.ai understands this. They offer customizable annotation solutions that align with the specific goals and challenges faced by each client, allowing for a tailored approach to data labeling.
Best Practices for Effective Image Annotation
To maximize the benefits of image annotation for machine learning, follow these best practices:
1. Clearly Define Objectives
Before starting the annotation process, define clear objectives. Understand what you aim to achieve with your machine learning model, as this will guide the annotation strategy.
2. Choose the Right Annotation Tool
Investing in the right annotation tool is critical. Evaluate different platforms, such as the ones offered by KeyLabs.ai, before committing to ensure they meet your needs.
3. Train Annotators
Provide training for your annotation team. Proper training ensures that annotators understand the guidelines and maintain consistency across the data.
4. Implement Quality Checks
Regular quality checks during the annotation process can catch errors early on. This proactive approach saves time and resources in the long run.
5. Foster Collaboration
Encourage collaboration among team members. Leveraging diverse insights can enhance the quality and relevancy of image annotations.
Conclusion
As businesses increasingly adopt image annotation for machine learning, they unlock the potential of AI to drive growth and efficiency. Platforms like KeyLabs.ai offer comprehensive solutions that empower organizations to harness the power of their visual data effectively. By implementing the right strategies and leveraging the appropriate tools, your business can stay at the forefront of innovation and achieve a competitive advantage in a rapidly evolving market.
Investing in image annotation is investing in the future of your organization. Start your journey with KeyLabs.ai today and transform how you interact with data.