The Impact of Malware Machine Learning on Business Security
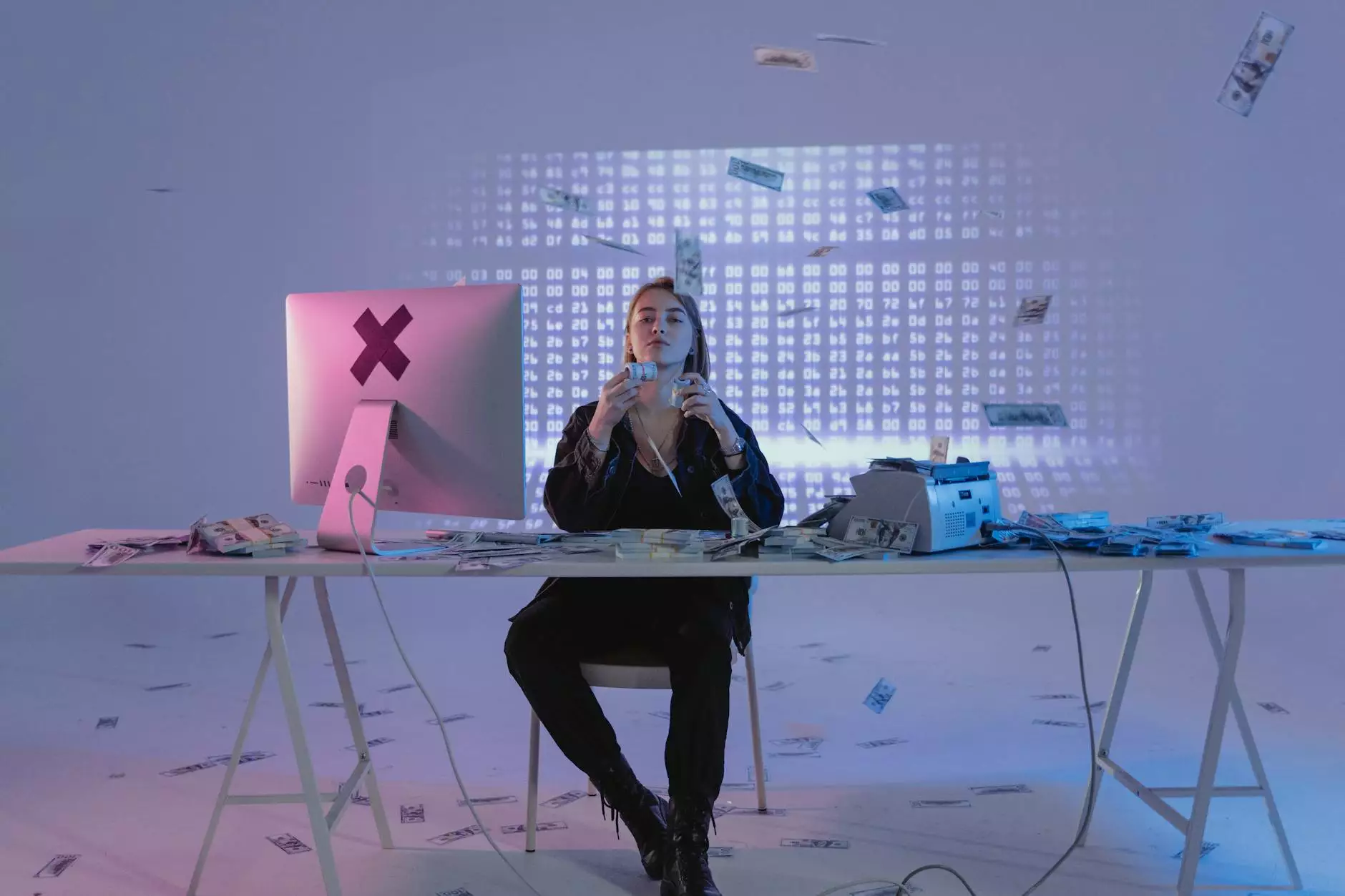
In today's rapidly evolving digital landscape, businesses face an increasing number of cyber threats, making robust security measures more crucial than ever. As organizations strive to protect their sensitive data and maintain the integrity of their IT systems, innovative technologies such as malware machine learning are proving to be game-changers in the realm of cybersecurity. This article delves deep into how malware machine learning can significantly bolster business security and IT services, ensuring businesses like Spambrella remain resilient against potential threats.
Understanding Malware Machine Learning
Malware machine learning represents the intersection of cybersecurity and artificial intelligence, specifically designed to analyze vast amounts of data in order to identify and mitigate malware threats. Machine learning algorithms are adept at recognizing patterns and anomalies in data, which can be applied to improve malware detection techniques.
Machine learning models learn from historical cybersecurity incidents, adjusting their detection mechanisms based on what they have learned. This allows organizations to proactively identify potential threats and respond swiftly, minimizing damage and breaches.
How Malware Machine Learning Enhances Business Security
The integration of machine learning in cybersecurity frameworks provides several key benefits to businesses:
- Proactive Threat Detection: Unlike traditional security measures that rely on signature-based detection, machine learning can identify new and evolving malware variants through behavioral analysis.
- Reduced False Positives: Machine learning systems continuously improve their accuracy, significantly reducing the number of false positives, ensuring that businesses aren't inundated with unnecessary alerts.
- Automated Response: Advanced machine learning models can automate threat responses, allowing organizations to respond to incidents in real-time without human intervention.
- Scalability: As businesses grow, machine learning models can scale effortlessly to manage increasing amounts of data without a corresponding increase in resource allocation.
The Role of Machine Learning in Malware Detection
Machine learning improves malware detection through various techniques and methodologies:
1. Anomaly Detection
Anomaly detection involves identifying deviations from the norm in user and system activities. By learning what constitutes "normal" behavior for users and devices, machine learning models can flag unusual behavior that may indicate a malware infection or data breach.
2. Feature Extraction
This method focuses on extracting important features from malware samples to classify them accurately. Machine learning algorithms assess characteristics—such as file size, coding structures, and the execution environment—to determine whether a file is malicious.
3. Classification
Classification algorithms categorize malware into types based on their features. By utilizing labeled datasets, these models can improve their accuracy and become adept at identifying previously unseen malware variants.
Why Businesses Should Invest in Malware Machine Learning Solutions
Investing in malware machine learning solutions is essential for businesses looking to establish robust cybersecurity measures. The benefits of these systems extend beyond mere threat detection:
- Cost Efficiency: The cost of a data breach can be astronomical. By preventing incidents through early detection and automated responses, companies can save significant sums associated with recovery efforts.
- Reputation Management: A strong cybersecurity posture enhances customer trust and confidence. Businesses known for effectively managing cyber threats are more likely to attract and retain clients.
- Compliance and Regulation: Many industries are subject to regulations regarding data privacy and protection. Implementing machine learning in malware detection helps businesses meet compliance requirements.
- Future-Proofing: With the rise of sophisticated cyber threats, investing in advanced technologies like machine learning positions businesses effectively to combat emerging threats.
Challenges and Considerations in Implementing Malware Machine Learning
While the benefits are substantial, businesses must also consider the challenges involved in implementing malware machine learning solutions:
1. Data Quality
Effective machine learning models depend heavily on high-quality data. Businesses need to ensure they have access to clean, well-structured data sets to train their models effectively.
2. Skill Gaps
The implementation of machine learning for cybersecurity may require specialized skills. Organizations should invest in training existing staff or hiring experts with experience in both machine learning and cybersecurity.
3. Integration with Existing Systems
Integrating new machine learning solutions with legacy security systems can be complex. Organizations must plan carefully to ensure seamless functionality across all platforms.
4. Evolving Threat Landscape
The threat landscape is constantly evolving, and machine learning models must be updated regularly to adapt to new types of malware and attack vectors.
Conclusion
As businesses navigate a world filled with complex cybersecurity threats, malware machine learning stands out as a powerful tool to enhance security, streamline IT services, and safeguard sensitive information. By proactively detecting threats, reducing false positives, and automating responses, machine learning ensures that businesses like Spambrella remain equipped to face the challenges ahead.
Investing in these advanced technologies not only mitigates risks but also fosters a culture of security awareness and resilience within organizations. As machine learning continues to evolve, it will undoubtedly play a pivotal role in shaping the future of cybersecurity. Now is the time for businesses to embrace this technology and fortify their defenses against the growing threat of malware.